Calling Bullshit: The Art of Skepticism in a Data-Driven World (Penguin Random House, 2020) is a much-needed guide for folks awash in numbers who are just trying to make informed decisions. Anyone working or advocating in transportation should read it.
The preface begins with a definition of bullshit and a discussion of its different types. The authors, Carl T. Bergstrom and Jevin D. West, a biologist and a data scientist at the University of Washington, distinguish between old-school and new-school bullshit. Their book focusses on the new-school type which “uses the language of math and science and statistics to create the impression of rigor and accuracy,” and they introduce the concept of “mathiness,” their analog to comedian Stephen Colbert’s “truthiness.” Mathiness refers “to formulas and expressions that may look and feel like math—even as they disregard the logical coherence and formal rigor of actual mathematics.”
Each chapter guides the reader through topics like causality, selection bias, data visualization, and big data, with examples from contemporary news. The result is a topical, fast-paced and laugh-out-loud funny book.
Pernicious Percentages
As I was reading, however, I found myself providing my own examples from transportation. Take traffic enforcement. The statistic that approximately 30% of shootings of police happen during traffic stops has been cited in prominent court cases for decades and has been used to justify giving the police broad authority to search during a traffic stop.
Advertisement
But a student of Calling Bullshit will realize the more pertinent question takes shootings out of the denominator and puts it in the numerator: out of the total number of traffic stops, what percentage result in shootings of officers? A recent study of traffic stops in Florida showed that traffic stops are less dangerous than the 30% statistic would lead one to believe:
Under a conservative estimate, the rate for a felonious killing of an officer during a routine traffic stop was only 1 in every 6.5 million stops, the rate for an assault resulting in serious injury to an officer was only 1 in every 361,111 stops, and the rate for an assault against officers (whether it results in injury or not) was only 1 in every 6,959 stops.
Although those odds still might not be good enough to call policing a safe occupation, they leave a different impression than the 30% statistic.
Black Boxes
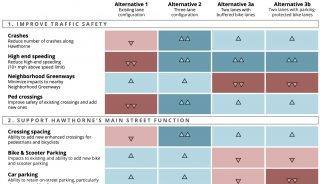
One of my favorite sections of the book was “Bullshit and Black Boxes.” A “black box” is a formula or device into which data is fed and results exit; but figuring out what actually happens in the box is either beyond your skillset or would take a lot of time. Whenever I look at one of the many Portland Bureau of Transportation prioritization schemes, even though they are well-documented, I feel like I’m in the presence of a black box. Luckily, Bergstrom and West advise the reader how to approach one — you don’t need to open it, just look critically at the input and the output.
The black box I bumped into a couple of years ago was the PedPDX Pedestrian Priority Network. I think this prioritization is worth taking time to understand, in that it follows a method which will most likely continue to be used (the Safe Routes to Schools project list, for example, uses a similar model). My calculations were made two years ago, and do not reflect more recent tweaks that might have been made to the data sets or rubrics.
PedPDX prioritizes streets to receive safety improvements with this formula:
Safety + Equity + Demand = Priority Score.
Although it looks fair enough, be wary of an equation that takes that form—the policy jiggering takes place at the back end with the scoring rubric used to build the Safety, Equity and Demand data sets. The scoring rubric in turn determines the number of points in each set, and their distribution. This is important because the shape of the data sets (the distribution) can deform the results into something far from the even-steven contributions the headline equation implies.
Did I lose you in the previous paragraph? I told you it was a black box!
More specifically, PedPDX designed the safety rubric to select for the characteristics of high crash corridors. To accomplish this, the scoring rubric initially gave almost two thirds of Portland’s streets a pedestrian risk score of 0 (that lowest score has since been updated from 0 to 1, out of 10 points). Thus the Safety data set skewed far to the left and on average did not contribute much to the final results. Perhaps counter-intuitively, this gave the few streets that did receive a high safety score a big leg up. The output distortion away from the “equally weighted” top line equation is quite big. For example, 2,702 streets received a top score of 10 in the equity category, but only eight street segments in the safety category received a 10.
The important point is that this method is vulnerable to distortion which is difficult to discover without access to the databases and a spreadsheet. Thus it is not transparent, and the seemingly even safety, equity, demand equation can end up being misleading.
But back to the book! I highly recommend Calling Bullshit. The authors are wonderful teachers and do an outstanding job of explaining some difficult concepts. Reading it will make you a more discerning consumer of data, and might even embolden you to take a look under the hood yourself. As Bergstrom and West write, “You do not need to be a professional statistician or econometrician or data scientist to think critically about quantitative arguments, nor do you need extensive data sets and weeks of effort to see through bullshit. It is often sufficient to apply basic logical reasoning to a problem…”
(This might be a little rosy, sometimes you do need a data set, a spreadsheet and a week!)
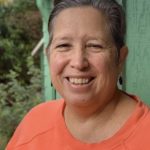
— Lisa Caballero, lisacaballero853@gmail.com
— Get our headlines delivered to your inbox.
— Support this independent community media outlet with a one-time contribution or monthly subscription.